May 05, 2019 Open-Source Psychometrics Project ( home about) Holland Code (RIASEC) Test. This is an interactive version of the IIP RIASEC Markers. The Holland Occupational Themes is a theory of personality that focuses on career and vocational choice. It groups people on the basis of their suitability for six different categories of occupations. Psychometrics is a field of study concerned with the theory and technique of psychological measurement.As defined by the US National Council on Measurement in Education (NCME), psychometrics refers to psychological measurement. Generally, it refers to the field in psychology and education that is devoted to testing, measurement, assessment, and related activities.
- Concurrent Validity Vs Predictive Validity
- Reliability Psychometrics
- Psychometric Scales
- Validity In Psychometrics Pdf Format
- Validity In Psychometrics Pdf Sample
Part of a series on |
Psychology |
---|
|
Psychometrics is a field of study concerned with the theory and technique of psychologicalmeasurement. As defined by the US National Council on Measurement in Education (NCME), psychometrics refers to psychological measurement. Generally, it refers to the field in psychology and education that is devoted to testing, measurement, assessment, and related activities.[1]
The field is concerned with the objective measurement of skills and knowledge, abilities, attitudes, personality traits, and educational achievement. Some psychometric researchers focus on the construction and validation of assessment instruments such as questionnaires, tests, raters' judgments, and personality tests. Others focus on research relating to measurement theory (e.g., item response theory; intraclass correlation).
Practitioners are described as psychometricians. Psychometricians usually possess a specific qualification, and most are psychologists with advanced graduate training. In addition to traditional academic institutions, many psychometricians work for the government or in human resources departments. Others specialize as learning and development professionals.
- 1Historical foundation
- 4Theoretical approaches
- 5Standards of quality
- 8References
Historical foundation[edit]
Psychological testing has come from two streams of thought: the first, from Darwin, Galton, and Cattell on the measurement of individual differences, and the second, from Herbart, Weber, Fechner, and Wundt and their psychophysical measurements of a similar construct. The second set of individuals and their research is what has led to the development of experimental psychology, and standardized testing.[2]
Victorian stream[edit]
Charles Darwin was the inspiration behind Sir Francis Galton who led to the creation of psychometrics. In 1859, Darwin published his book 'The Origin of Species', which pertained to individual differences in animals. This book discussed how individual members in a species differ and how they possess characteristics that are more adaptive and successful or less adaptive and less successful. Those who are adaptive and successful are the ones that survive and give way to the next generation, who would be just as or more adaptive and successful. This idea, studied previously in animals, led to Galton's interest and study of human beings and how they differ one from another, and more importantly, how to measure those differences.
Galton wrote a book entitled 'Hereditary Genius' about different characteristics that people possess and how those characteristics make them more 'fit' than others. Today these differences, such as sensory and motor functioning (reaction time, visual acuity, and physical strength) are important domains of scientific psychology. Much of the early theoretical and applied work in psychometrics was undertaken in an attempt to measure intelligence. Galton, often referred to as 'the father of psychometrics,' devised and included mental tests among his anthropometric measures. James McKeen Cattell, who is considered a pioneer of psychometrics went on to extend Galton's work. Cattell also coined the term mental test, and is responsible for the research and knowledge which ultimately led to the development of modern tests. (Kaplan & Saccuzzo, 2010)
German stream[edit]
The origin of psychometrics also has connections to the related field of psychophysics. Around the same time that Darwin, Galton, and Cattell were making their discoveries, Herbart was also interested in 'unlocking the mysteries of human consciousness' through the scientific method. (Kaplan & Saccuzzo, 2010) Herbart was responsible for creating mathematical models of the mind, which were influential in educational practices in years to come.
E.H. Weber built upon Herbart's work and tried to prove the existence of a psychological threshold, saying that a minimum stimulus was necessary to activate a sensory system. After Weber, G.T. Fechner expanded upon the knowledge he gleaned from Herbart and Weber, to devise the law that the strength of a sensation grows as the logarithm of the stimulus intensity. A follower of Weber and Fechner, Wilhelm Wundt is credited with founding the science of psychology. It is Wundt's influence that paved the way for others to develop psychological testing.[2]
20th century[edit]
The psychometrician L. L. Thurstone, founder and first president of the Psychometric Society in 1936, developed and applied a theoretical approach to measurement referred to as the law of comparative judgment, an approach that has close connections to the psychophysical theory of Ernst Heinrich Weber and Gustav Fechner. In addition, Spearman and Thurstone both made important contributions to the theory and application of factor analysis, a statistical method developed and used extensively in psychometrics.[citation needed] In the late 1950s, Leopold Szondi made an historical and epistemological assessment of the impact of statistical thinking onto psychology during previous few decades: 'in the last decades, the specifically psychological thinking has been almost completely suppressed and removed, and replaced by a statistical thinking. Precisely here we see the cancer of testology and testomania of today.'[3]
More recently, psychometric theory has been applied in the measurement of personality, attitudes, and beliefs, and academic achievement. Measurement of these unobservable phenomena is difficult, and much of the research and accumulated science in this discipline has been developed in an attempt to properly define and quantify such phenomena. Critics, including practitioners in the physical sciences and social activists, have argued that such definition and quantification is impossibly difficult, and that such measurements are often misused, such as with psychometric personality tests used in employment procedures:
- 'For example, an employer wanting someone for a role requiring consistent attention to repetitive detail will probably not want to give that job to someone who is very creative and gets bored easily.'[4]
Figures who made significant contributions to psychometrics include Karl Pearson, Henry F. Kaiser, Carl Brigham, L. L. Thurstone, Anne Anastasi, Georg Rasch, Eugene Galanter, Johnson O'Connor, Frederic M. Lord, Ledyard R Tucker, Arthur Jensen, and David Andrich.
Definition of measurement in the social sciences[edit]
The definition of measurement in the social sciences has a long history. A currently widespread definition, proposed by Stanley Smith Stevens (1946), is that measurement is 'the assignment of numerals to objects or events according to some rule.' This definition was introduced in the paper in which Stevens proposed four levels of measurement. Although widely adopted, this definition differs in important respects from the more classical definition of measurement adopted in the physical sciences, namely that scientific measurement entails 'the estimation or discovery of the ratio of some magnitude of a quantitative attribute to a unit of the same attribute' (p. 358)[5]
Indeed, Stevens's definition of measurement was put forward in response to the British Ferguson Committee, whose chair, A. Ferguson, was a physicist. The committee was appointed in 1932 by the British Association for the Advancement of Science to investigate the possibility of quantitatively estimating sensory events. Although its chair and other members were physicists, the committee also included several psychologists. The committee's report highlighted the importance of the definition of measurement. While Stevens's response was to propose a new definition, which has had considerable influence in the field, this was by no means the only response to the report. Another, notably different, response was to accept the classical definition, as reflected in the following statement:
- Measurement in psychology and physics are in no sense different. Physicists can measure when they can find the operations by which they may meet the necessary criteria; psychologists have but to do the same. They need not worry about the mysterious differences between the meaning of measurement in the two sciences. (Reese, 1943, p. 49)
Concurrent Validity Vs Predictive Validity
These divergent responses are reflected in alternative approaches to measurement. For example, methods based on covariance matrices are typically employed on the premise that numbers, such as raw scores derived from assessments, are measurements. Such approaches implicitly entail Stevens's definition of measurement, which requires only that numbers are assigned according to some rule. The main research task, then, is generally considered to be the discovery of associations between scores, and of factors posited to underlie such associations.[citation needed]
On the other hand, when measurement models such as the Rasch model are employed, numbers are not assigned based on a rule. Instead, in keeping with Reese's statement above, specific criteria for measurement are stated, and the goal is to construct procedures or operations that provide data that meet the relevant criteria. Measurements are estimated based on the models, and tests are conducted to ascertain whether the relevant criteria have been met.[citation needed]
Instruments and procedures[edit]
The first[citation needed]psychometric instruments were designed to measure the concept of intelligence.[6] One historical approach involved the Stanford-Binet IQ test, developed originally by the French psychologist Alfred Binet. Intelligence tests are useful tools for various purposes. An alternative conception of intelligence is that cognitive capacities within individuals are a manifestation of a general component, or general intelligence factor, as well as cognitive capacity specific to a given domain.[citation needed]
Another major focus in psychometrics has been on personality testing. There have been a range of theoretical approaches to conceptualizing and measuring personality. Some of the better known instruments include the Minnesota Multiphasic Personality Inventory, the Five-Factor Model (or 'Big 5') and tools such as Personality and Preference Inventory and the Myers-Briggs Type Indicator. Attitudes have also been studied extensively using psychometric approaches.[citation needed] A common method in the measurement of attitudes is the use of the Likert scale. An alternative method involves the application of unfolding measurement models, the most general being the Hyperbolic Cosine Model (Andrich & Luo, 1993).[7]
Theoretical approaches[edit]
Psychometricians have developed a number of different measurement theories. These include classical test theory (CTT) and item response theory (IRT).[8][9] An approach which seems mathematically to be similar to IRT but also quite distinctive, in terms of its origins and features, is represented by the Rasch model for measurement. The development of the Rasch model, and the broader class of models to which it belongs, was explicitly founded on requirements of measurement in the physical sciences.[10]
Psychometricians have also developed methods for working with large matrices of correlations and covariances. Techniques in this general tradition include: factor analysis,[11] a method of determining the underlying dimensions of data; multidimensional scaling,[12] a method for finding a simple representation for data with a large number of latent dimensions; and data clustering, an approach to finding objects that are like each other. All these multivariate descriptive methods try to distill large amounts of data into simpler structures. More recently, structural equation modeling[13] and path analysis represent more sophisticated approaches to working with large covariance matrices. These methods allow statistically sophisticated models to be fitted to data and tested to determine if they are adequate fits.
One of the main deficiencies in various factor analyses is a lack of consensus in cutting points for determining the number of latent factors. A usual procedure is to stop factoring when eigenvalues drop below one because the original sphere shrinks. The lack of the cutting points concerns other multivariate methods, also.[citation needed]
Key concepts[edit]
Key concepts in classical test theory are reliability and validity. A reliable measure is one that measures a construct consistently across time, individuals, and situations. A valid measure is one that measures what it is intended to measure. Reliability is necessary, but not sufficient, for validity.
Both reliability and validity can be assessed statistically. Consistency over repeated measures of the same test can be assessed with the Pearson correlation coefficient, and is often called test-retest reliability.[14] Similarly, the equivalence of different versions of the same measure can be indexed by a Pearson correlation, and is called equivalent forms reliability or a similar term.[14]
Internal consistency, which addresses the homogeneity of a single test form, may be assessed by correlating performance on two halves of a test, which is termed split-half reliability; the value of this Pearson product-moment correlation coefficient for two half-tests is adjusted with the Spearman–Brown prediction formula to correspond to the correlation between two full-length tests.[14] Perhaps the most commonly used index of reliability is Cronbach's α, which is equivalent to the mean of all possible split-half coefficients. Other approaches include the intra-class correlation, which is the ratio of variance of measurements of a given target to the variance of all targets.
There are a number of different forms of validity. Criterion-related validity can be assessed by correlating a measure with a criterion measure theoretically expected to be related. When the criterion measure is collected at the same time as the measure being validated the goal is to establish concurrent validity; when the criterion is collected later the goal is to establish predictive validity. A measure has construct validity if it is related to measures of other constructs as required by theory. Content validity is a demonstration that the items of a test do an adequate job of covering the domain being measured. In a personnel selection example, test content is based on a defined statement or set of statements of knowledge, skill, ability, or other characteristics obtained from a job analysis.
Item response theory models the relationship between latent traits and responses to test items. Among other advantages, IRT provides a basis for obtaining an estimate of the location of a test-taker on a given latent trait as well as the standard error of measurement of that location. For example, a university student's knowledge of history can be deduced from his or her score on a university test and then be compared reliably with a high school student's knowledge deduced from a less difficult test. Scores derived by classical test theory do not have this characteristic, and assessment of actual ability (rather than ability relative to other test-takers) must be assessed by comparing scores to those of a 'norm group' randomly selected from the population. In fact, all measures derived from classical test theory are dependent on the sample tested, while, in principle, those derived from item response theory are not.
Many psychometricians are also concerned with finding and eliminating test bias from their psychological tests. Test bias is a form of systematic (i.e., non-random) error which leads to examinees from one demographic group having an unwarranted advantage over examinees from another demographic group.[15] According to leading experts, test bias may cause differences in average scores across demographic groups, but differences in group scores are not sufficient evidence that test bias is actually present because the test could be measuring real differences among groups.[16][15] Psychometricians use sophisticated scientific methods to search for test bias and eliminate it. Research shows that it is usually impossible for people reading a test item to accurately determine whether it is biased or not.[17]
Standards of quality[edit]
The considerations of validity and reliability typically are viewed as essential elements for determining the quality of any test. However, professional and practitioner associations frequently have placed these concerns within broader contexts when developing standards and making overall judgments about the quality of any test as a whole within a given context. A consideration of concern in many applied research settings is whether or not the metric of a given psychological inventory is meaningful or arbitrary.[18]
Testing standards[edit]
In 2014, the American Educational Research Association (AERA), American Psychological Association (APA), and National Council on Measurement in Education (NCME) published a revision of the Standards for Educational and Psychological Testing,[19] which describes standards for test development, evaluation, and use. The Standards cover essential topics in testing including validity, reliability/errors of measurement, and fairness in testing. The book also establishes standards related to testing operations including test design and development, scores, scales, norms, score linking, cut scores, test administration, scoring, reporting, score interpretation, test documentation, and rights and responsibilities of test takers and test users. Finally, the Standards cover topics related to testing applications, including psychological testing and assessment, workplace testing and credentialing, educational testing and assessment, and testing in program evaluation and public policy.
Evaluation standards[edit]
In the field of evaluation, and in particular educational evaluation, the Joint Committee on Standards for Educational Evaluation[20] has published three sets of standards for evaluations. The Personnel Evaluation Standards[21] was published in 1988, The Program Evaluation Standards (2nd edition)[22] was published in 1994, and The Student Evaluation Standards[23] was published in 2003.
Each publication presents and elaborates a set of standards for use in a variety of educational settings. The standards provide guidelines for designing, implementing, assessing and improving the identified form of evaluation.[24] Each of the standards has been placed in one of four fundamental categories to promote educational evaluations that are proper, useful, feasible, and accurate. In these sets of standards, validity and reliability considerations are covered under the accuracy topic. For example, the student accuracy standards help ensure that student evaluations will provide sound, accurate, and credible information about student learning and performance.
Non-human: animals and machines[edit]
Psychometrics addresses human abilities, attitudes, traits and educational evolution. Notably, the study of behavior, mental processes and abilities of non-human animals is usually addressed by comparative psychology, or with a continuum between non-human animals and the rest of animals by evolutionary psychology. Nonetheless there are some advocators for a more gradual transition between the approach taken for humans and the approach taken for (non-human) animals.[25][26][27][28]
The evaluation of abilities, traits and learning evolution of machines has been mostly unrelated to the case of humans and non-human animals, with specific approaches in the area of artificial intelligence. A more integrated approach, under the name of universal psychometrics, has also been proposed.[29]
See also[edit]
References[edit]
Bibliography[edit]
- Andrich, D. & Luo, G. (1993). 'A hyperbolic cosine model for unfolding dichotomous single-stimulus responses'(PDF). Applied Psychological Measurement. 17 (3): 253–276. CiteSeerX10.1.1.1003.8107. doi:10.1177/014662169301700307.[permanent dead link]
- Michell, J. (1999). Measurement in Psychology. Cambridge: Cambridge University Press. DOI: 10.1017/CBO9780511490040
- Rasch, G. (1960/1980). Probabilistic models for some intelligence and attainment tests. Copenhagen, Danish Institute for Educational Research), expanded edition (1980) with foreword and afterword by B.D. Wright. Chicago: The University of Chicago Press.
- Reese, T.W. (1943). 'The application of the theory of physical measurement to the measurement of psychological magnitudes, with three experimental examples'. Psychological Monographs. 55: 1–89.
- Stevens, S. S. (1946). 'On the theory of scales of measurement'. Science. 103 (2684): 677–80. Bibcode:1946Sci...103..677S. doi:10.1126/science.103.2684.677. PMID17750512.
- Thurstone, L.L. (1927). 'A law of comparative judgement'. Psychological Review. 34 (4): 278–286. doi:10.1037/h0070288.
- Thurstone, L.L. (1929). The Measurement of Psychological Value. In T.V. Smith and W.K. Wright (Eds.), Essays in Philosophy by Seventeen Doctors of Philosophy of the University of Chicago. Chicago: Open Court.
- Thurstone, L.L. (1959). The Measurement of Values. Chicago: The University of Chicago Press.
- S.F. Blinkhorn (1997). 'Past imperfect, future conditional: fifty years of test theory'. Br. J. Math. Statist. Psychol. 50 (2): 175–185. doi:10.1111/j.2044-8317.1997.tb01139.x.
Notes[edit]
- ^National Council on Measurement in Education http://www.ncme.org/ncme/NCME/Resource_Center/Glossary/NCME/Resource_Center/Glossary1.aspx?hkey=4bb87415-44dc-4088-9ed9-e8515326a061#anchorPArchived 2017-07-22 at the Wayback Machine
- ^ abKaplan, R.M., & Saccuzzo, D.P. (2010). Psychological Testing: Principles, Applications, and Issues. (8th ed.). Belmont, CA: Wadsworth, Cengage Learning.
- ^Leopold Szondi (1960) Das zweite Buch: Lehrbuch der Experimentellen Triebdiagnostik. Huber, Bern und Stuttgart, 2nd edition. Ch.27, From the Spanish translation, B)II Las condiciones estadisticas, p.396. Quotation:
el pensamiento psicologico especifico, en las ultima decadas, fue suprimido y eliminado casi totalmente, siendo sustituido por un pensamiento estadistico. Precisamente aqui vemos el cáncer de la testología y testomania de hoy.
- ^Psychometric Assessments. Psychometric Assessments . University of Melbourne.
- ^Michell, Joel (August 1997). 'Quantitative science and the definition of measurement in psychology'. British Journal of Psychology. 88 (3): 355–383. doi:10.1111/j.2044-8295.1997.tb02641.x.
- ^'Los diferentes tipos de tests psicometricos - examen psicometrico'. examenpsicometrico.com.
- ^Andrich, D. & Luo, G. (1993). A hyperbolic cosine latent trait model for unfolding dichotomous single-stimulus responses. Applied Psychological Measurement, 17, 253-276.
- ^Embretson, S.E., & Reise, S.P. (2000). Item Response Theory for Psychologists. Mahwah, NJ: Erlbaum.
- ^Hambleton, R.K., & Swaminathan, H. (1985). Item Response Theory: Principles and Applications. Boston: Kluwer-Nijhoff.
- ^Rasch, G. (1960/1980). Probabilistic models for some intelligence and attainment tests. Copenhagen, Danish Institute for Educational Research, expanded edition (1980) with foreword and afterword by B.D. Wright. Chicago: The University of Chicago Press.
- ^Thompson, B.R. (2004). Exploratory and Confirmatory Factor Analysis: Understanding Concepts and Applications. American Psychological Association.
- ^Davison, M.L. (1992). Multidimensional Scaling. Krieger.
- ^Kaplan, D. (2008). Structural Equation Modeling: Foundations and Extensions, 2nd ed. Sage.
- ^ abc'Home - Educational Research Basics by Del Siegle'. www.gifted.uconn.edu.
- ^ abWarne, Russell T.; Yoon, Myeongsun; Price, Chris J. (2014). 'Exploring the various interpretations of 'test bias''. Cultural Diversity and Ethnic Minority Psychology. 20 (4): 570–582. doi:10.1037/a0036503. PMID25313435.
- ^Reynolds, C. R. (2000). Why is psychometric research on bias in mental testing so often ignored? Psychology, Public Policy, and Law, 6, 144-150. doi:10.1037/1076-8971.6.1.144
- ^Reschly, D. J. (1980) Psychological evidence in the Larry P. opinion: A case of right problem-wrong solution? School Psychology Review, 9, 123-125.
- ^Blanton, H., & Jaccard, J. (2006). Arbitrary metrics in psychology.Archived 2006-05-10 at the Wayback MachineAmerican Psychologist, 61(1), 27-41.
- ^'The Standards for Educational and Psychological Testing'. http://www.apa.org.External link in
|website=
(help) - ^Joint Committee on Standards for Educational EvaluationArchived 2009-10-15 at the Wayback Machine
- ^Joint Committee on Standards for Educational Evaluation. (1988). The Personnel Evaluation Standards: How to Assess Systems for Evaluating Educators.Archived 2005-12-12 at the Wayback Machine Newbury Park, CA: Sage Publications.
- ^Joint Committee on Standards for Educational Evaluation. (1994). The Program Evaluation Standards, 2nd Edition.Archived 2006-02-22 at the Wayback Machine Newbury Park, CA: Sage Publications.
- ^Committee on Standards for Educational Evaluation. (2003). The Student Evaluation Standards: How to Improve Evaluations of Students.Archived 2006-05-24 at the Wayback Machine Newbury Park, CA: Corwin Press.
- ^[E. Cabrera-Nguyen. 'Author guidelines for reporting scale development and validation results in the Journal of the Society for Social Work and Research]'. Academia.edu. 1 (2): 99–103.
- ^Humphreys, L.G. (1987). 'Psychometrics considerations in the evaluation of intraspecies differences in intelligence'. Behav Brain Sci. 10 (4): 668–669. doi:10.1017/s0140525x0005514x.
- ^Eysenck, H.J. (1987). 'The several meanings of intelligence'. Behav Brain Sci. 10 (4): 663. doi:10.1017/s0140525x00055060.
- ^Locurto, C. & Scanlon, C (1987). 'Individual differences and spatial learning factor in two strains of mice'. Behav Brain Sci. 112: 344–352.
- ^King, James E & Figueredo, Aurelio Jose (1997). 'The five-factor model plus dominance in chimpanzee personality'. Journal of Research in Personality. 31 (2): 257–271. doi:10.1006/jrpe.1997.2179.
- ^J. Hernández-Orallo; D.L. Dowe; M.V. Hernández-Lloreda (2013). 'Universal Psychometrics: Measuring Cognitive Abilities in the Machine Kingdom'(PDF). Cognitive Systems Research. 27: 50–74. doi:10.1016/j.cogsys.2013.06.001. hdl:10251/50244.
Further reading[edit]
- Robert F. DeVellis (2016). Scale Development: Theory and Applications. SAGE Publications. ISBN978-1-5063-4158-3.
- Borsboom, Denny (2005). Measuring the Mind: Conceptual Issues in Contemporary Psychometrics. Cambridge: Cambridge University Press. ISBN978-0-521-84463-5. Lay summary (28 June 2010).
- Leslie A. Miller; Robert L. Lovler (2015). Foundations of Psychological Testing: A Practical Approach. SAGE Publications. ISBN978-1-4833-6927-3.
- Roderick P. McDonald (2013). Test Theory: A Unified Treatment. Psychology Press. ISBN978-1-135-67530-1.
- Paul Kline (2000). The Handbook of Psychological Testing. Psychology Press. ISBN978-0-415-21158-1.
- Rush AJ Jr; First MB; Blacker D (2008). Handbook of Psychiatric Measures. American Psychiatric Publishing. ISBN978-1-58562-218-4. OCLC85885343.
- Ann C Silverlake (2016). Comprehending Test Manuals: A Guide and Workbook. Taylor & Francis. ISBN978-1-351-97086-0.
- Fenton H (2019). 'Top 10 Tips on how to prepare for a psychometric test to get that job!'. Business Optimization Training Institute.
- Dr. Snigdha Rai (2018). 'An Ultimate Guide to Psychometric Tests'. Mercer Mettl.
External links[edit]
Wikiversity has learning resources about Psychometrics |
Look up psychometrics in Wiktionary, the free dictionary. |
- The Psychometrics Centre, University of Cambridge[1]
Library resources about psychometrics |
- ^Sanford, David (18 November 2017). 'Cambridge just told me Big Data doesn't work yet'. LinkedIn.[dead link]
Validity is the extent to which a concept,[1] conclusion or measurement is well-founded and likely corresponds accurately to the real world. The word 'valid' is derived from the Latin validus, meaning strong. The validity of a measurement tool (for example, a test in education) is the degree to which the tool measures what it claims to measure. Validity is based on the strength of a collection of different types of evidence (e.g. face validity, construct validity, etc.) described in greater detail below.

In psychometrics, validity has a particular application known as test validity: 'the degree to which evidence and theory support the interpretations of test scores' ('as entailed by proposed uses of tests').[2]
It is generally accepted that the concept of scientific validity addresses the nature of reality in terms of statistical measures and as such is an epistemological and philosophical issue as well as a question of measurement. The use of the term in logic is narrower, relating to the truth of inferences made from premises. In logic, and therefore as the term is applied to any epistemological claim, validity refers to the consistency of an argument flowing from the premises to the conclusion; as such, the truth of the claim in logic is not only reliant on validity. Rather, an argumentative claim is true if and only if it is both valid and sound. This means the argument flows without contradiction from the premises or the conclusion, and all of the premises and the conclusion correspond to known facts. As such, 'scientific or statistical validity' is not a deductive claim that is necessarily truth preserving, but is an inductive claim that remains true or false in an undecided manner. This is why 'scientific or statistical validity' is a claim that is qualified as being either strong or weak in its nature, it is never necessary nor certainly true. This has the effect of making claims of 'scientific or statistical validity' open to interpretation as to what, in fact, the facts of the matter mean.
Validity is important because it can help determine what types of tests to use, and help to make sure researchers are using methods that are not only ethical, and cost-effective, but also a method that truly measures the idea or constructs in question.
- 1Test validity
- 1.3Content validity
- 1.4Criterion validity
- 2Experimental validity
- 2.3External validity
Test validity[edit]
Validity (accuracy) [edit]
Validity[3] of an assessment is the degree to which it measures what it is supposed to measure. This is not the same as reliability, which is the extent to which a measurement gives results that are very consistent. Within validity, the measurement does not always have to be similar, as it does in reliability. However, just because a measure is reliable, it is not necessarily valid. E.g. a scale that is 5 pounds off is reliable but not valid. A test cannot be valid unless it is reliable. Validity is also dependent on the measurement measuring what it was designed to measure, and not something else instead.[4] Validity (similar to reliability) is a relative concept; validity is not an all-or-nothing idea. There are many different types of validity.
Construct validity[edit]
Construct validity refers to the extent to which operationalizations of a construct (e.g., practical tests developed from a theory) measure a construct as defined by a theory. It subsumes all other types of validity. For example, the extent to which a test measures intelligence is a question of construct validity. A measure of intelligence presumes, among other things, that the measure is associated with things it should be associated with (convergent validity), not associated with things it should not be associated with (discriminant validity).[5]
Construct validity evidence involves the empirical and theoretical support for the interpretation of the construct. Such lines of evidence include statistical analyses of the internal structure of the test including the relationships between responses to different test items. They also include relationships between the test and measures of other constructs. As currently understood, construct validity is not distinct from the support for the substantive theory of the construct that the test is designed to measure. As such, experiments designed to reveal aspects of the causal role of the construct also contribute to constructing validity evidence.[5]
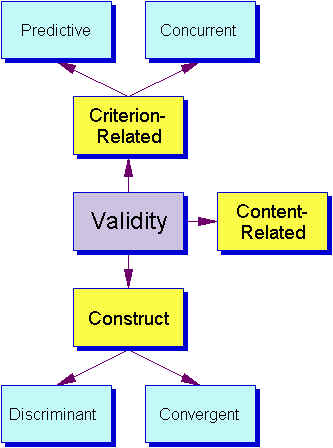
Content validity[edit]
Content validity is a non-statistical type of validity that involves 'the systematic examination of the test content to determine whether it covers a representative sample of the behavior domain to be measured' (Anastasi & Urbina, 1997 p. 114). For example, does an IQ questionnaire have items covering all areas of intelligence discussed in the scientific literature?
Content validity evidence involves the degree to which the content of the test matches a content domain associated with the construct. For example, a test of the ability to add two numbers should include a range of combinations of digits. A test with only one-digit numbers, or only even numbers, would not have good coverage of the content domain. Content related evidence typically involves a subject matter expert (SME) evaluating test items against the test specifications. Before going to the final administration of questionnaires, the researcher should consult the validity of items against each of the constructs or variables and accordingly modify measurement instruments on the basis of SME's opinion.
A test has content validity built into it by careful selection of which items to include (Anastasi & Urbina, 1997). Items are chosen so that they comply with the test specification which is drawn up through a thorough examination of the subject domain. Foxcroft, Paterson, le Roux & Herbst (2004, p. 49)[6] note that by using a panel of experts to review the test specifications and the selection of items the content validity of a test can be improved. The experts will be able to review the items and comment on whether the items cover a representative sample of the behavior domain.
Face validity[edit]
Face validity is an estimate of whether a test appears to measure a certain criterion; it does not guarantee that the test actually measures phenomena in that domain. Measures may have high validity, but when the test does not appear to be measuring what it is, it has low face validity. Indeed, when a test is subject to faking (malingering), low face validity might make the test more valid. Considering one may get more honest answers with lower face validity, it is sometimes important to make it appear as though there is low face validity whilst administering the measures.
Face validity is very closely related to content validity. While content validity depends on a theoretical basis for assuming if a test is assessing all domains of a certain criterion (e.g. does assessing addition skills yield in a good measure for mathematical skills? To answer this you have to know, what different kinds of arithmetic skills mathematical skills include) face validity relates to whether a test appears to be a good measure or not. This judgment is made on the 'face' of the test, thus it can also be judged by the amateur.
Face validity is a starting point, but should never be assumed to be probably valid for any given purpose, as the 'experts' have been wrong before—the Malleus Malificarum (Hammer of Witches) had no support for its conclusions other than the self-imagined competence of two 'experts' in 'witchcraft detection,' yet it was used as a 'test' to condemn and burn at the stake tens of thousands men and women as 'witches.'[7]
Criterion validity[edit]
Criterion validity evidence involves the correlation between the test and a criterion variable (or variables) taken as representative of the construct. In other words, it compares the test with other measures or outcomes (the criteria) already held to be valid. For example, employee selection tests are often validated against measures of job performance (the criterion), and IQ tests are often validated against measures of academic performance (the criterion).
If the test data and criterion data are collected at the same time, this is referred to as concurrent validity evidence. If the test data are collected first in order to predict criterion data collected at a later point in time, then this is referred to as predictive validity evidence.
Concurrent validity[edit]
Concurrent validity refers to the degree to which the operationalization correlates with other measures of the same construct that are measured at the same time. When the measure is compared to another measure of the same type, they will be related (or correlated). Returning to the selection test example, this would mean that the tests are administered to current employees and then correlated with their scores on performance reviews.
Predictive validity[edit]
Predictive validity refers to the degree to which the operationalization can predict (or correlate with) other measures of the same construct that are measured at some time in the future. Again, with the selection test example, this would mean that the tests are administered to applicants, all applicants are hired, their performance is reviewed at a later time, and then their scores on the two measures are correlated.
This is also when measurement predicts a relationship between what is measured and something else; predicting whether or not the other thing will happen in the future. High correlation between ex-ante predicted and ex-post actual outcomes is the strongest proof of validity.
Experimental validity[edit]
The validity of the design of experimental research studies is a fundamental part of the scientific method, and a concern of research ethics. Without a valid design, valid scientific conclusions cannot be drawn.
Statistical conclusion validity[edit]
Statistical conclusion validity is the degree to which conclusions about the relationship among variables based on the data are correct or ‘reasonable’. This began as being solely about whether the statistical conclusion about the relationship of the variables was correct, but now there is a movement towards moving to ‘reasonable’ conclusions that use: quantitative, statistical, and qualitative data.[8]
Statistical conclusion validity involves ensuring the use of adequate sampling procedures, appropriate statistical tests, and reliable measurement procedures.[9] As this type of validity is concerned solely with the relationship that is found among variables, the relationship may be solely a correlation.
Internal validity[edit]
Internal validity is an inductive estimate of the degree to which conclusions about causal relationships can be made (e.g. cause and effect), based on the measures used, the research setting, and the whole research design. Good experimental techniques, in which the effect of an independent variable on a dependent variable is studied under highly controlled conditions, usually allow for higher degrees of internal validity than, for example, single-case designs.
Eight kinds of confounding variable can interfere with internal validity (i.e. with the attempt to isolate causal relationships):
- History, the specific events occurring between the first and second measurements in addition to the experimental variables
- Maturation, processes within the participants as a function of the passage of time (not specific to particular events), e.g., growing older, hungrier, more tired, and so on.
- Testing, the effects of taking a test upon the scores of a second testing.
- Instrumentation, changes in calibration of a measurement tool or changes in the observers or scorers may produce changes in the obtained measurements.
- Statistical regression, operating where groups have been selected on the basis of their extreme scores.
- Selection, biases resulting from differential selection of respondents for the comparison groups.
- Experimental mortality, or differential loss of respondents from the comparison groups.
- Selection-maturation interaction, etc. e.g., in multiple-group quasi-experimental designs
External validity[edit]
External validity concerns the extent to which the (internally valid) results of a study can be held to be true for other cases, for example to different people, places or times. In other words, it is about whether findings can be validly generalized. If the same research study was conducted in those other cases, would it get the same results?
A major factor in this is whether the study sample (e.g. the research participants) are representative of the general population along relevant dimensions. Other factors jeopardizing external validity are:
- Reactive or interaction effect of testing, a pretest might increase the scores on a posttest
- Interaction effects of selection biases and the experimental variable.
- Reactive effects of experimental arrangements, which would preclude generalization about the effect of the experimental variable upon persons being exposed to it in non-experimental settings
- Multiple-treatment interference, where effects of earlier treatments are not erasable.
Ecological validity[edit]
Ecological validity is the extent to which research results can be applied to real-life situations outside of research settings. This issue is closely related to external validity but covers the question of to what degree experimental findings mirror what can be observed in the real world (ecology = the science of interaction between organism and its environment). To be ecologically valid, the methods, materials and setting of a study must approximate the real-life situation that is under investigation.
Ecological validity is partly related to the issue of experiment versus observation. Typically in science, there are two domains of research: observational (passive) and experimental (active). The purpose of experimental designs is to test causality, so that you can infer A causes B or B causes A. But sometimes, ethical and/or methological restrictions prevent you from conducting an experiment (e.g. how does isolation influence a child's cognitive functioning?). Then you can still do research, but it is not causal, it is correlational. You can only conclude that A occurs together with B. Both techniques have their strengths and weaknesses.
Relationship to internal validity[edit]
Reliability Psychometrics
On first glance, internal and external validity seem to contradict each other – to get an experimental design you have to control for all interfering variables. That is why you often conduct your experiment in a laboratory setting. While gaining internal validity (excluding interfering variables by keeping them constant) you lose ecological or external validity because you establish an artificial laboratory setting. On the other hand, with observational research you can not control for interfering variables (low internal validity) but you can measure in the natural (ecological) environment, at the place where behavior normally occurs. However, in doing so, you sacrifice internal validity.
The apparent contradiction of internal validity and external validity is, however, only superficial. The question of whether results from a particular study generalize to other people, places or times arises only when one follows an inductivist research strategy. If the goal of a study is to deductively test a theory, one is only concerned with factors which might undermine the rigor of the study, i.e. threats to internal validity.
Diagnostic validity[edit]
In psychiatry there is a particular issue with assessing the validity of the diagnostic categories themselves. In this context:[10]
- content validity may refer to symptoms and diagnostic criteria;
- concurrent validity may be defined by various correlates or markers, and perhaps also treatment response;
- predictive validity may refer mainly to diagnostic stability over time;
- discriminant validity may involve delimitation from other disorders.
Robins and Guze proposed in 1970 what were to become influential formal criteria for establishing the validity of psychiatric diagnoses. They listed five criteria:[10]
- distinct clinical description (including symptom profiles, demographic characteristics, and typical precipitants)
- laboratory studies (including psychological tests, radiology and postmortem findings)
- delimitation from other disorders (by means of exclusion criteria)
- follow-up studies showing a characteristic course (including evidence of diagnostic stability)
- family studies showing familial clustering
These were incorporated into the Feighner Criteria and Research Diagnostic Criteria that have since formed the basis of the DSM and ICD classification systems.

Kendler in 1980 distinguished between:[10]
- antecedent validators (familial aggregation, premorbid personality, and precipitating factors)
- concurrent validators (including psychological tests)
- predictive validators (diagnostic consistency over time, rates of relapse and recovery, and response to treatment)
Nancy Andreasen (1995) listed several additional validators – molecular genetics and molecular biology, neurochemistry, neuroanatomy, neurophysiology, and cognitive neuroscience – that are all potentially capable of linking symptoms and diagnoses to their neural substrates.[10]
Kendell and Jablinsky (2003) emphasized the importance of distinguishing between validity and utility, and argued that diagnostic categories defined by their syndromes should be regarded as valid only if they have been shown to be discrete entities with natural boundaries that separate them from other disorders.[10]
Kendler (2006) emphasized that to be useful, a validating criterion must be sensitive enough to validate most syndromes that are true disorders, while also being specific enough to invalidate most syndromes that are not true disorders. On this basis, he argues that a Robins and Guze criterion of 'runs in the family' is inadequately specific because most human psychological and physical traits would qualify - for example, an arbitrary syndrome comprising a mixture of 'height over 6 ft, red hair, and a large nose' will be found to 'run in families' and be 'hereditary', but this should not be considered evidence that it is a disorder. Kendler has further suggested that 'essentialist' gene models of psychiatric disorders, and the hope that we will be able to validate categorical psychiatric diagnoses by 'carving nature at its joints' solely as a result of gene discovery, are implausible.[11]
In the United States Federal Court System validity and reliability of evidence is evaluated using the Daubert Standard: see Daubert v. Merrell Dow Pharmaceuticals. Perri and Lichtenwald (2010) provide a starting point for a discussion about a wide range of reliability and validity topics in their analysis of a wrongful murder conviction.[12]
See also[edit]
References[edit]
Psychometric Scales
- ^Brains, Willnat, Manheim, Rich 2011. Empirical Political Analysis 8th edition. Boston, MA: Longman p. 105
- ^American Educational Research Association, Psychological Association, & National Council on Measurement in Education. (1999). Standards for Educational and Psychological Testing. Washington, DC: American Educational Research Association.
- ^ National Council on Measurement in Education. http://www.ncme.org/ncme/NCME/Resource_Center/Glossary/NCME/Resource_Center/Glossary1.aspx?hkey=4bb87415-44dc-4088-9ed9-e8515326a061#anchorV
- ^Kramer, Geoffrey P., Douglas A. Bernstein, and Vicky Phares. Introduction to clinical psychology. 7th ed. Upper Saddle River, NJ: Pearson Prentice Hall, 2009. Print.
- ^ abCronbach, Lee J.; Meehl, Paul E. (1955). 'Construct validity in psychological tests'. Psychological Bulletin. 52 (4): 281–302. doi:10.1037/h0040957. ISSN0033-2909. PMID13245896.
- ^Foxcroft, C., Paterson, H., le Roux, N., & Herbst, D. Human Sciences Research Council, (2004). 'Psychological assessment in South Africa: A needs analysis: The test use patterns and needs of psychological assessment practitioners: Final Report: July. Retrieved from website: http://www.hsrc.ac.za/research/output/outputDocuments/1716_Foxcroft_Psychologicalassessmentin%20SA.pdf
- ^The most common estimates are between 40,000 and 60,000 deaths. Brian Levack (The Witch Hunt in Early Modern Europe) multiplied the number of known European witch trials by the average rate of conviction and execution, to arrive at a figure of around 60,000 deaths. Anne Lewellyn Barstow (Witchcraze) adjusted Levack's estimate to account for lost records, estimating 100,000 deaths. Ronald Hutton (Triumph of the Moon) argues that Levack's estimate had already been adjusted for these, and revises the figure to approximately 40,000.
- ^Cozby, Paul C.. Methods in behavioral research. 10th ed. Boston: McGraw-Hill Higher Education, 2009. Print.
- ^Jonathan Javid (6 November 2015). 'Measurement validity and reliability'. slideshare.net. Retrieved 23 March 2018.
- ^ abcdeKendell, R; Jablensky, A (2003). 'Distinguishing between the validity and utility of psychiatric diagnoses'. The American Journal of Psychiatry. 160 (1): 4–12. doi:10.1176/appi.ajp.160.1.4. PMID12505793.
- ^Kendler, KS (2006). 'Reflections on the relationship between psychiatric genetics and psychiatric nosology'. The American Journal of Psychiatry. 163 (7): 1138–46. doi:10.1176/appi.ajp.163.7.1138. PMID16816216.
- ^Perri, FS; Lichtenwald, TG (2010). 'The Precarious Use Of Forensic Psychology As Evidence: The Timothy Masters Case'(PDF). Champion Magazine (July): 34–45.
Further reading[edit]
Validity In Psychometrics Pdf Format
- Cronbach, L. J.; Meehl, P. E. (1955), 'Construct validity in psychological tests', Psychological Bulletin, 52 (4): 281–302, doi:10.1037/h0040957, PMID13245896
- Rupp, A. A.; Pant, H. A. (2007), 'Validity theory', in Salkind, Neil J. (ed.), Encyclopedia of Measurement and Statistics, SAGE Publishing
Wikiversity has learning resources about Validity |